SORBA Advanced Industrial AI Platform Improving OEE
Within the manufacturing industry, manufacturers are constantly looking to optimize production within factories to improve efficiency and reduce cost. This has opened new opportunities in the constant drive for optimization in real-time with Edge computing combined with Cloud services, such as the Industrial Internet of Things (IIoT), data lakes, analytics, and machine learning (ML). SORBA enables manufacturers that are executing their digital transformation and integrating operational technologies (OT) and information technology (IT) with the application of machine learning which is relatively a new concept.
Introduction into SORBA-ML
This document provides an introduction into SORBA-ML machine learning and how it can be used in manufacturing by providing example use cases of optimizing production through the variable of Overall Equipment Effectiveness (OEE).
Across discrete and process manufacturing, one of the main metrics used to gauge manufacturing productivity is Overall Equipment Effectiveness (OEE). SORBA-PPA (Production Performance Analytics) provides real-time OEE, a percentage that represents the productivity of a plant, production line or machine. The OEE percentage is made up of the percentages of quality, throughput or performance, and the availability or non-stoppage time as shown in the following formula:

SORBA-ML is used to focus on the factors that influence the variables of availability, performance, and quality and can improve OEE. It is generally accepted that OEE greater than 85% is considered world class, with most manufacturers operating in the 60% range.
SORBA-ML can measure and reduce downtime (improve availability), reduce defects (improve quality), and improve the productivity of a factory, production line, or machine, resulting in increasing OEE.
SORBA-ML used to monitor and control the 3 OEE variables
To accomplish this, SORBA-ML uses process and production data to measure and analyze the manufacturing processes so that insights can be drawn for improvements. SORBA-SDE collects manufacturing operational data from factory machines, sensor data, operational MES (Manufacturing Execution System) data, maintenance records from asset management systems and production planning data from the ERP (Enterprise Resource Planning) systems. SORBA-ML correlates, analyzes, and trains the machine learning models for anomaly detection and generates predictions which can then be used to monitor and control the three OEE variables.
Automating Manufacturing Processes
SORBA-ML APO (Advanced Process Optimization) & APC (Advanced Process Control) applies machine learning models to the manufacturing process where processes can be automated. SORBA-ML enables manufacturers to optimize their physical manufacturing process through the predictions of the machine learning models.
Below is an example of a real-time SORBA-PPA OEE Dashboard collecting data and generating the 3 main production KPIs Performance, Availability & Quality for a large co-packer of pet food.
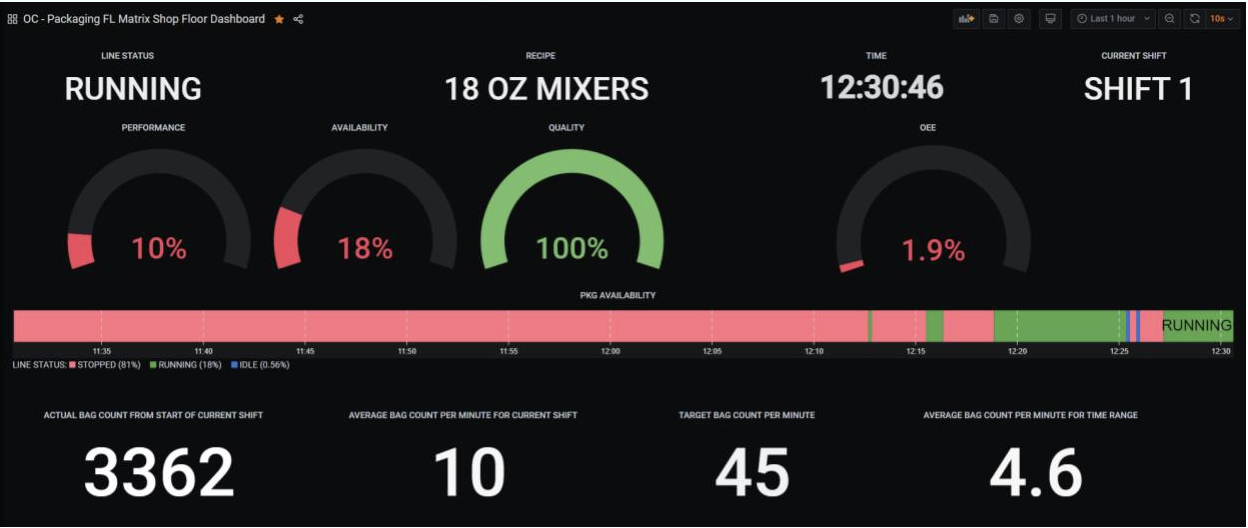
The current snapshot of the customer’s performance dashboard demonstrates poor results and contains a high potential for improvement.
The customer’s dashboard displays a quality metric of 100%. This was artificially set to pass all products as good because no automated quality control inspection was implemented. All inspection is performed by humans and quality data has not included in the SORBA-PPA software.
How to Use AI to Improve Availability?
SORBA-ML addresses all KPIs and learns good production behavior. To improve availability, the dependency of equipment reliability is the key. SORBA-ML trains anomaly detection models and deploys them to the SORBA-SDE edge processing platform. SORBA trained the models to generate real-time predictions referred to as SORBOTS. Anomaly detection SORBOTS for conveyor motors that move product in the packaging cell are trained to alert operators of an abnormal behavior that could result in downtime and lower availability. The early anomaly warning enables operations to be proactive and prevent a downtime event resulting in poor OEE.
How to Use AI to Improve Performance?
For the co-packer to improve performance they need to increase yield and production rate based on the ideal production rate baseline of the packaging line. The typical action would be to increase the line speed which could have the opposite result. To achieve the objective of increasing the production rate, SORBA-APC is used to determine the optimal setpoint speed of the packaging line that will result in maximizing production rate increased by 5-10%. SORBA-APC applies machine learning and deep learning to build digital twin of the process and determine the optimal high and low setpoint range. SORBA-APC automates the process by automatically adjusting the line speed that does not require operator intervention.
How to Use AI to Improve Quality?
For quality improvement since the data is manually collected and a human determines “PASS” or “FAIL”, SORBA-ML trains a model to classify a good quality product. This is referred to as a virtual sensor or virtual quality inspector. The PASS/FAIL data is setup as a response variable to the SORBA-ML model and using the real-time process data such as, speed, temperature, flow and pressure is correlated to the quality test generating an automatic inspection. To achieve better quality inspection results processing images with SORBA-ML can classify good quality products using CNN (convolutional neural networks).
Using Digital Twin Technology
For operational planning, the SORBA-ML application creates a simulation or digit twin and forecasting models for manufacturing process and demand planning. SORBA digital twins simulates the process determining required capacity to improve cycle time reducing production line interruptions.
SORBA-ML operational forecasting models provide forward planning on labor and materials to support next month of manufacturing. Other SORBA-ML use cases that impact operational planning is supply chain as an input can provide variance to a production planning schedule.
Use Case: Global Beverage Producer
The following use case is a global beverage producer that is using SORBA-ML to generate predictions for production schedules.
The current situation before SORBA-ML was implemented, the customer was experiencing complexities in the configuration with lots of manual inputs, speed and performance issues and low accuracy with their current prediction models. In addition to operational improvement, the customer was seeking to decrease carbon emissions and generate energy saving through optimization production planning from (4) main datasets, production volume, consumption of electrical power, heat and water, production hours and CO2. A SORBA-ML model was trained to predict a production plan with data from SAP, MES and QCS systems integrated with the SORBA-SDE workflow management for automatic data ingestion.
The result was a model that can learn and adapt to varying conditions with no manual inputs required. A SORBA-IIoT cloud-based solution was used across multiple sites to build models trained to reduce water, CO2, heat, and user dashboard for operations to visualize predicted ideal production rate.
SORBA-ML enabled smart MES adding intelligence to legacy systems improving overall effectiveness.
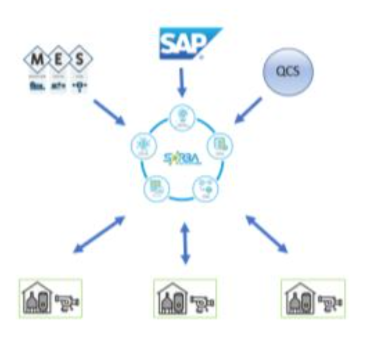