Understanding Advanced Process Optimization & Control (APC) with SORBA.ai
Manufacturing processes are complex and dynamic, involving multiple variables that impact product quality, production yield, energy consumption, and overall operational efficiency. Traditional control methods often fall short in optimizing these processes due to their inability to adapt to changing conditions and complex interactions. This is where APC comes into play, leveraging the power of AI and ML to unlock the full potential of manufacturing operations.
So, what is an APC? Advanced Process Optimization & Control (APC) is an AI and ML-based technology that optimizes industrial processes by monitoring their performance and behavior in real-time. The technology employs AI and ML algorithms to analyze process data, identify patterns and trends, and adjust process parameters in real-time to optimize performance. It involves the integration of data from various sources, such as sensors, production systems, and external factors, to continuously analyze, predict, and optimize process performance.
The key components of APC include:
- Data Acquisition: APC relies on data as its foundation. Data is collected from various sources, such as sensors, SCADA (Supervisory Control and Data Acquisition) systems, and other industrial data systems, to provide real-time insights into the process performance. SORBA has over 30 industrial drivers to allow you to connect with any data source you may want to bring in to your APC solution.
- Data Analytics: Advanced data analytics techniques, such as AI and ML, are applied to process data to uncover hidden patterns, trends, and correlations. These insights are used to build predictive models and generate actionable recommendations for process optimization.
- Process Optimization: APC uses the insights from data analytics to optimize process parameters in real-time, making adjustments to control inputs, setpoints, and other operational parameters to maximize production efficiency, reduce waste, and improve product quality.
- Closed-Loop Control: APC enables closed-loop control, where real-time data and analytics are used to continuously monitor and adjust process parameters to maintain optimal performance. This closed-loop feedback mechanism ensures that the process remains optimized even as conditions change.
- Visualization and Reporting: APC provides intuitive visualization and reporting tools that allow engineers to monitor and analyze process performance in real-time. This helps in identifying bottlenecks, diagnosing issues, and making data-driven decisions for process improvement.
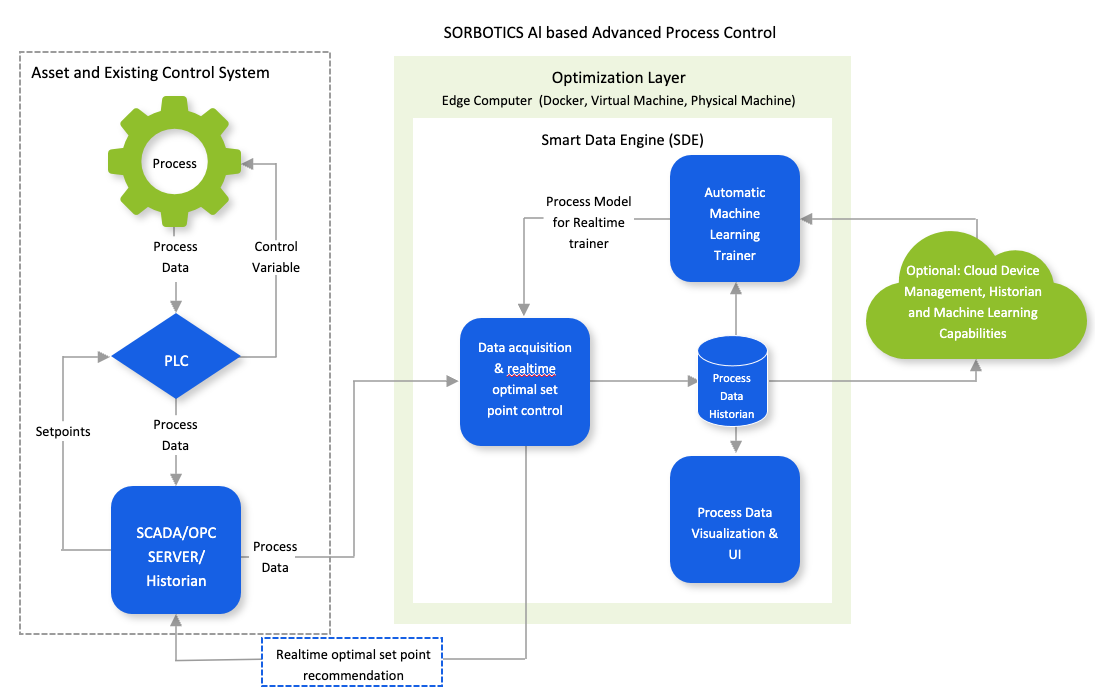
What are the benefits of APC?
- Increased Efficiency: APC identifies optimal process conditions, reducing energy consumption, minimizing waste, and maximizing production yield. This results in significant cost savings and increased operational efficiency.
- Improved Product Quality: APC monitors and controls process parameters in real-time, ensuring that products meet desired specifications consistently. This leads to improved product quality and reduced variability, resulting in higher customer satisfaction.
- Enhanced Operational Flexibility: APC enables adaptive and flexible manufacturing processes that can respond to changing market demands, process variability, and external factors. This allows manufacturers to adapt quickly and efficiently to changing conditions. APC can also increase operational flexibility by freeing up operators to complete other tasks as more setpoints are controlled by SORBA.
- Better Decision Making: SORBA’s APC solution uses the historical data from the machine to ensure that the best operating decision is being made for the given operating conditions. Setpoints can be controlled dynamically in order to keep things working at an optimal level.
APC and SORBA.ai
Our advanced AI software platform combines data acquisition, data analytics, process optimization, closed-loop control, and visualization tools to enable APC for industrial engineers. With SORBA, you can use the Machine Learning Trainer to create an optimization algorithm based on your historical data for the machine. All you need to do after acquiring your data is determine your control variables, optimization variable and goal, and at least one independent variable. These terms refer to:
- Control variables: These are the variables that you want SORBA to take control over in order to complete the optimization.
- Optimization variable and goal: This refers to the variable on your system that you are either trying to maximize or minimize. For instance, you could be trying to maximize a variable representative of your energy savings or representative of some efficiency metric. Or you may want to minimize the amount of time a certain process takes.
- Independent variable: This refers to a variable that you want to remain unaffected by the optimization process. This variable provides a frame of reference for the operating conditions of the machine and ensures that the optimization doesn’t interfere with core requirements of the process you are optimizing.
With these variables identified, you can easily use SORBA.ai to analyze a historical dataset, generate a machine learning algorithm, and deploy that setting into a real time environment so that you can start to see improvements in the process you want to optimize.
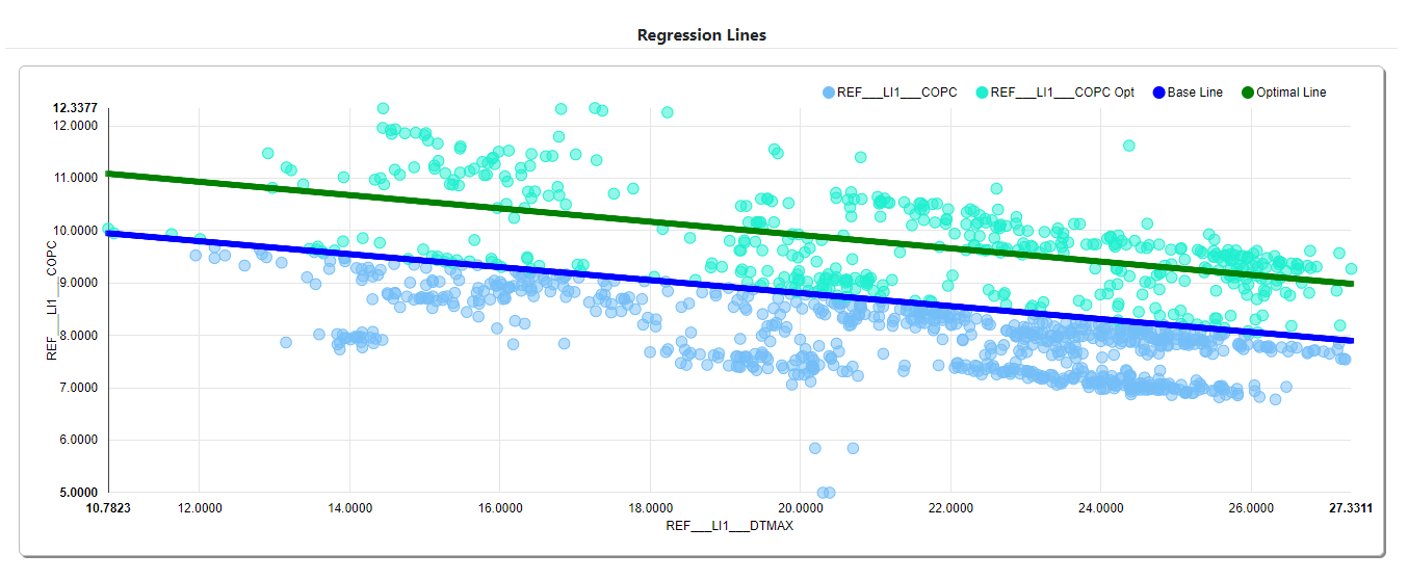