In the fast-paced and competitive world of industrial manufacturing, efficiency and productivity are paramount to success. To achieve optimal performance, organizations are turning to process control and optimization as essential tools in their arsenal. In this blog post, tailored specifically for industrial manufacturers, we will delve into the critical aspects of process control and optimization: who should leverage it, what it entails, when it should be employed, where it can be implemented, why it is a game-changer, and how it works.
Who should use optimization?
Optimization algorithms are indispensable for industrial manufacturing across various sectors and company sizes. Whether you operate a small-scale factory or a large-scale production facility, if your operations involve complex systems, data analysis, or decision-making processes, optimization can empower you to achieve enhanced efficiency, reduce costs, and optimize your manufacturing processes for better outcomes.
What is optimization in industrial manufacturing?
Optimization algorithms in industrial manufacturing, such as those offered by SORBA.ai, enable you to maximize the performance of your manufacturing processes. These algorithms operate by selecting specific variables and adjusting them to either maximize or minimize outcomes, ultimately driving your production towards predefined optimization goals. Optimization encompasses a broad range of applications, including energy savings, quality improvements, process speed enhancements, and more. By automating control over critical variables, optimization algorithms support operators in achieving better outcomes and streamlining manufacturing operations.
How optimization algorithms work:
Optimization algorithms in SORBA work by selecting a variable to either maximize or minimize, allowing users to have SORBA take over a set list of variables in order to achieve this optimization goal. Common uses for optimization algorithms include energy savings, improvement around some quality metric, and increased process speed. These algorithms can be used to assist operators by moving some setpoints to be automatically and dynamically controlled.
The first step to an optimization project is to identify and select variables. The variables for an optimization algorithm are:
Optimization variable and goal
Independent Variable or Variables
Control Variables
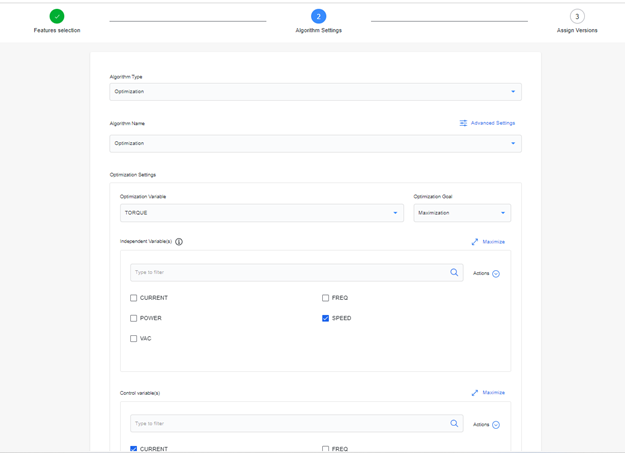
The optimization variable is the variable that the user wants the optimization algorithm to impact in some way. When this variable is selected, the user can also select whether they want to maximize or minimize this variable.
The independent variable is one of the most difficult elements of an optimization problem to identify. This is a variable that you want to remain unaffected by the optimization algorithm. You can select multiple independent variables when creating an optimization algorithm. This variable prevents a situation where a solution such as turning off the machine to save energy could be reached. This variable should also be representative of the different operating conditions for the machine. Some examples could include the speed of a conveyor system as you may want the speed of your process to be unaffected by the optimization. In refrigeration optimization projects a commonly used independent variable is the difference between the temperature of the coldest load and the ambient temperature since this represents the current weather as well as the demand on the refrigeration system, and you want to make sure that your energy optimization isn’t leaving the refrigeration system incapable of meeting the demand. In an ideal situation, the independent variable should have a relatively linear relationship with the optimization variable, but this is not always necessary.
The control variable or variables are the variables that you want SORBA to take control over. For each variable selected, once the model is deployed into a real time setting, values are output for each control variable in order to achieve the optimization goal. When configuring an optimization algorithm users will also see an additional variable section referred to as variables to keep by user. This simply refers to any tags that the user wants to be included in the final model even if the model determines that those tags do not have a significant impact on the optimization goal.
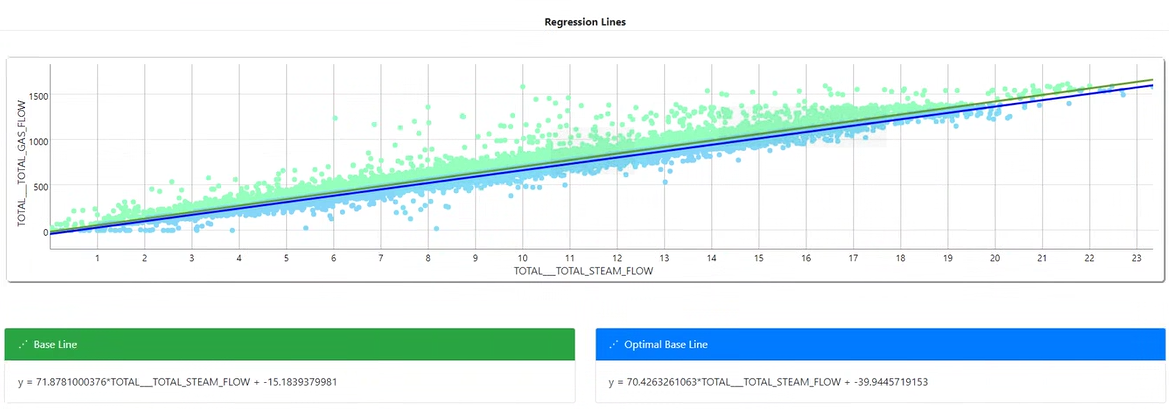
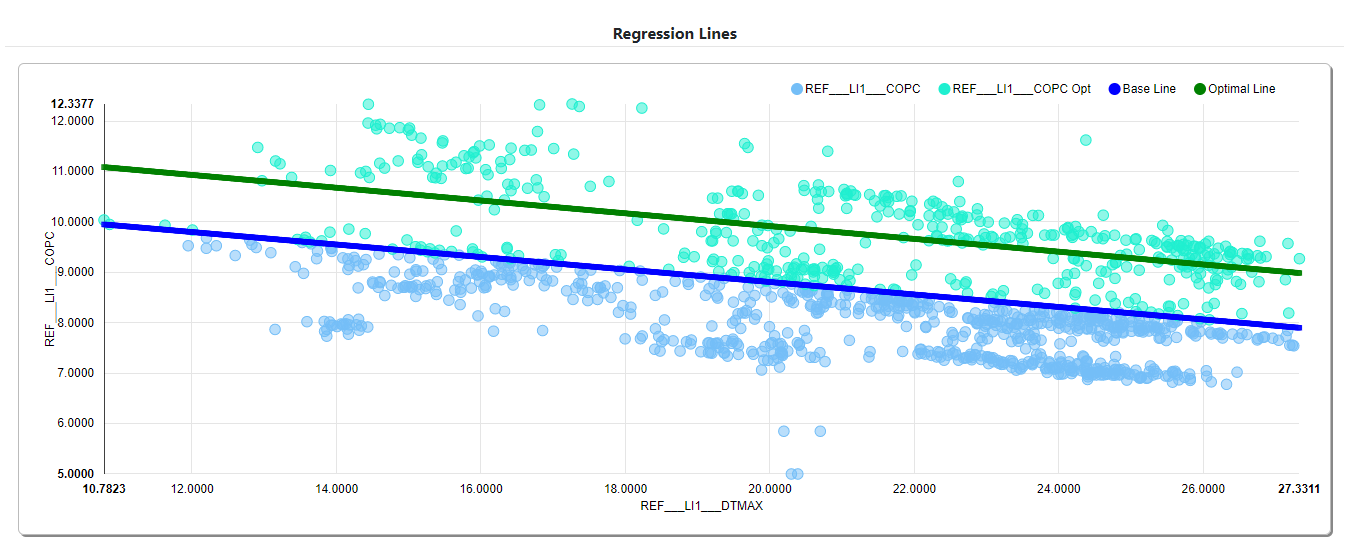
Now, the final step is to close the loop. Prior to closing the loop, most users will want to conduct an open loop test of the optimization algorithm. To do this, it is deployed into production but is not allowed to write values back to the system. This enables a user to validate the setpoints recommended by the algorithm. Once it is verified that the algorithm is producing reasonable setpoints, the loop can be closed. This means that the setpoint values can be written back to the machine so that SORBA can fully take control over that process in order to achieve the optimization goal in real time.
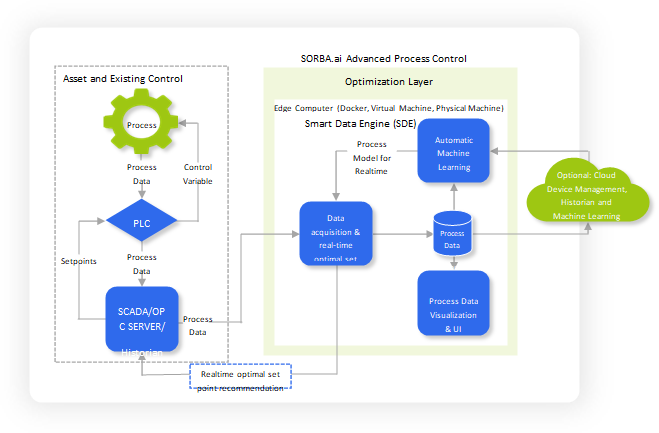
When should optimization be implemented in industrial manufacturing?
Optimization should be considered whenever you aim to enhance your manufacturing processes and extract maximum efficiency. Some key scenarios where optimization is particularly beneficial include:
- Process optimization: Optimize complex manufacturing processes to reduce waste, improve product quality, and enhance overall efficiency.
- Resource allocation: Optimize the allocation of resources, such as raw materials, energy, and labor, to minimize costs and maximize productivity.
- Supply chain optimization: Streamline supply chain operations by optimizing inventory levels, transportation routes, and production scheduling to minimize lead times and improve customer satisfaction.
- Equipment optimization: Fine-tune the operation of machinery and equipment to optimize performance, reduce downtime, and prolong their lifespan.
Where should optimization be implemented?
Optimization can be implemented across various areas in the industrial manufacturing landscape. Some potential applications include:
- Production lines: Optimize the workflow, sequencing, and configuration of production lines to maximize throughput and minimize bottlenecks.
- Energy management: Optimize energy consumption by identifying inefficiencies, optimizing equipment usage, and implementing energy-saving measures.
- Quality control: Implement optimization algorithms to continuously monitor and adjust quality parameters, ensuring consistent product quality and reducing defects.
- Maintenance planning: Optimize maintenance schedules based on equipment performance data and predictive analytics to minimize unplanned downtime and improve maintenance efficiency.
Why should optimization be leveraged?
By embracing optimization, companies can reduce energy consumption, minimize waste, decrease emissions, conserve resources, and contribute to a more sustainable future.
- Energy efficiency: Reduces energy consumption by optimizing equipment operations and power usage, contributing to energy conservation efforts.
- Waste reduction: Minimizes material waste and raw material consumption, promoting sustainable production practices.
- Emissions reduction: Minimizes greenhouse gas emissions and air pollutants by optimizing processes and combustion.
- Water conservation: Minimizes water usage and promotes efficient water management, conserving this valuable resource.
- Supply chain optimization: Reduces the carbon footprint associated with transportation and logistics, leading to lower emissions.
- Sustainable product design: Optimization considers environmental factors, such as recyclable materials and energy-efficient designs, promoting eco-friendly products.
How does optimization work in industrial manufacturing?
Optimization algorithms follow a systematic approach:
- Variable selection: Identify the key variables that influence the desired outcome, such as energy consumption, quality metrics, or production speed.
- Optimization goal: Define the optimization goal, whether it's maximizing or minimizing the selected variables, aligning with your specific manufacturing objectives.
- Independent variables: Identify independent variables that should remain unaffected by the optimization algorithm, ensuring the stability and reliability of your manufacturing processes.
- Control variables: Determine the variables that the optimization algorithm will control to achieve the desired optimization goals.
- Model deployment: Deploy the optimization model in real-time to output values for the control variables, enabling the system to work towards achieving the optimization goal.
- Closed-loop operation: Conduct thorough testing and validation of the optimization algorithm before closing the loop. Once verified, the algorithm takes full control over the manufacturing process, actively adjusting setpoints to optimize performance in real-time.
Conclusion:
Optimization algorithms offer immense potential for industrial manufacturing, enabling companies to achieve new levels of efficiency, cost savings, and product quality. By leveraging these algorithms in diverse applications, industrial manufacturers can gain a competitive edge and thrive in today's dynamic business landscape.